Managing Risk and Preventing Fraud with Graph Databases
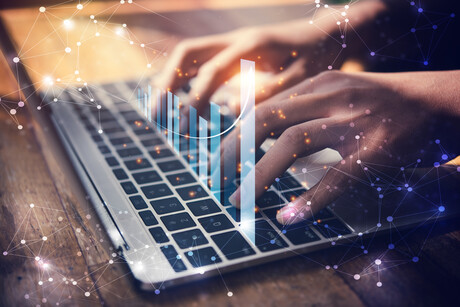
Ever since human beings began engaging in commercial activities, there has been fraud.
“What bothers me isn’t that fraud is not nice, or that fraud is mean. For fifteen thousand years, fraud and short-sighted thinking have never, ever worked. Not once. Eventually you get caught, things go south.” Mark Baum, The Big Short (2015).
Be it the earliest cons of passing off gold or silver mixed with base metals as pure, to the more elegant deceptions of Scottish adventurer Gregor McGregor, who sold fake government bonds for the non-existent Caribbean country of ‘Poyais’ to unwitting British investors in the early 18th Century — fraud has taken a myriad of forms and evolved as times have changed.
Today, fraudsters and money launderers operate predominantly in the digital world, where the ubiquitous use of credit cards and online transactions — while convenient for both consumers and retailers — has also deepened the complexity of business security and brought with it new risks for regulators and growth of organised crime.
Those risks have become a steadily growing and worrying problem in recent years. For example, LexisNexis reported that incidents of fraud amongst financial services firms increased dramatically over the last year, with instances of attempted fraud doubling and rates of fraud success increasing by an astonishing 85 percent over the same timeframe.
What this adds up to is a growing burden on consumers and business and new revenue for criminals, with estimates suggesting online payment fraud that has been tracked since 2018 will ultimately have a cost impact on merchants of over $130 billion by 2023.
And it’s not just retailers who are affected. Any financial services organisation that operates online — be they banks, insurers, mortgage lenders, investment and wealth fund managers, money exchange providers, even casinos and gaming companies — all can face the challenge of fraudsters in one way or another. The pressure is on for financial regulators and other relevant bodies to step up and counter this growing problem.
However, while online fraud methods may have become more sophisticated, so too has the technology to detect that fraud — and there is a new tool that has emerged which is enabling much more efficient and accurate identification of potentially unusual online transaction activity — the Graph Database.
Reliable Data is Key
What is common to all fraud is the fabrication of trust. When you trust in something or someone, you tend not to spend time thinking how they might be taking advantage of you. You go about your day feeling confident in the knowledge that you are in safe hands. All that time you spend not thinking about a fraudster is time they can use to carry out their deception and take your money.
However, the better you know someone you do business with, the harder it is for a fraudster to fabricate that trust. Knowing exactly who your clients and their associated entities are — and securing accurate data on them through an onboarding process — is vital for making that trust base rock solid.
Clearly, data governance, data ownership, and risk mitigation are all best practice activities for an organisation. But, without reliable data analysis, these activities are almost impossible to identify, report and control, especially when it comes to online transactions.
Tracking suspicious activity in online transactions is a tricky business — as it can be extremely challenging to efficiently identify unusual or unexpected activity and filter out any false positives.
However, the burgeoning technology of Graph Databases can enable the identification and investigation with the linking of data with sufficient speed and scale to recognise the patterns of fraudulent interactions, discover the hidden relationships within datasets and identify and distinguish the truly suspicious transactions in real time.
Recognising the risks in real time
In a typical online transaction, there are multiple points of connection between the people, the devices they use to make payments, and the credit cards and bank accounts that are accessed throughout the process.
The Australian Securities and Investments Commission (ASIC) provides examples of the kinds of suspicious activity that must be reported. This includes unusual or unexpected trading activity, trading ahead of a price-sensitive announcement, transactions that ‘make no economic sense’, instructions to place an order immediately or urgently, and orders inconsistent with previous behaviour or profile.
Without a technology that can analyse the deep links and connections between the many data points of financial processes and identify any that are suspicious for whatever reason, a fraudulent transaction may slip through the system at any moment and be approved.
Any technology tasked with spotting these moments of fraud must be capable of doing so across hundreds of thousands of data points — or more during peak times — and in the real-timeframe of milliseconds. If the technology can accurately spot fraud, it can stop fraud — and that’s what graph database technology does.
How TigerGraph delivers real-time fraud detection
As one of the fastest graph database analytics platforms available globally today, TigerGraph is built to provide the kinds of deep, ultra-quick analyses of large datasets that are needed for fraud detection and investigations. Whether related to online payments, dishonest business activities, identity theft, fake charities, debt collection, or even COVID-19 scams, TigerGraph is fast becoming a tool of preference for fraud identification across public and private sectors.
TigerGraph offers three advanced analytics capabilities to achieve this:
- Transactional or mutable data infrastructure — As payments are processed through a payment gateway, TigerGraph’s advanced analytics solution infrastructure can run queries that return multiple responses on the ‘hops’ between data points in sub-second time to verify that the connections indicate a pattern that is consistent with trustworthy transactions.
- Real-time deep link traversal — TigerGraph’s advanced analytics can examine deep ‘multi-hop’ connections to deliver responses on hundreds of thousands of payment history queries per second.
- Real-time computation and updates on the connected data — Sub-second response times for queries mean that TigerGraph’s system can evaluate a ‘trust score’ for a transaction, and flag it in real-time if it is inconsistent with previous behaviour, indicating fraud.
In a world that is doing business online at increasing volumes and speeds, a system that can enable regulators to identify and investigate fraud will build all the trust needed for a business and government authorities to succeed.
Contact Intech Solutions today for more information on Graph Databases, or to arrange a no obligation, free consultation. Telephone: (02) 8305 2100 or visit: https://www.intechsolutions.com.au.
The cyber battleground
Jake King, Director of Threat Intelligence at Elastic, talks with us about the evolving cyber...
Why trust is essential when delivering public services
When it comes to digital transformation within the public sector, nothing is more important than...
Taking control: Why organisations must protect passwords with a comprehensive password management strategy
Here are five steps that security teams looking to improve how they safeguard workforce...